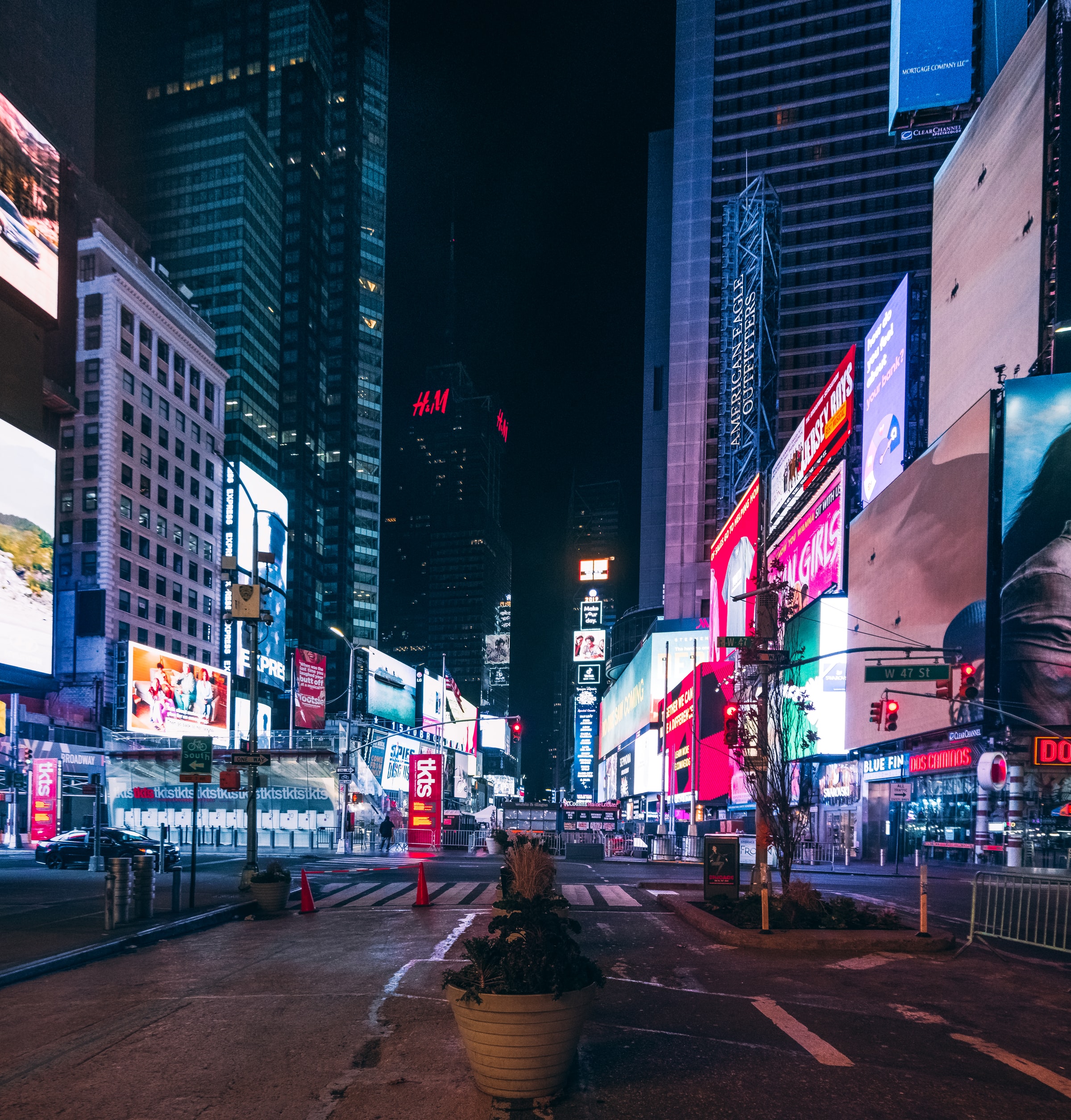
You can also read this post on Medium
At first glance, a post-pandemic economy might not seem the best time to invest in artificial intelligence. Most industries are hurting for revenue and cash flow. However, some firms will adopt AI to become more efficient and set the stage for growth after the economy recovers.
Firms that understand their business needs and AI use cases can make AI investments that achieve high ROI. AI is good at automating manual processes that require costly manpower. AI is also good at finding patterns in large datasets to generate insights. Used properly, AI can help companies cut costs and preserve revenue during the post-Coronavirus economic downturn.
While U.S. stocks staged a spectacular comeback as of June 2020, stocks don’t always reflect the business environment. Companies operate globally and countries in Asia and Latin America are hurting economically because of Covid-19. Future Covid waves could further harm business prospects. Savvy business leaders will explore how AI can help them navigate this environment.
Business Priorities will Dictate AI Strategy
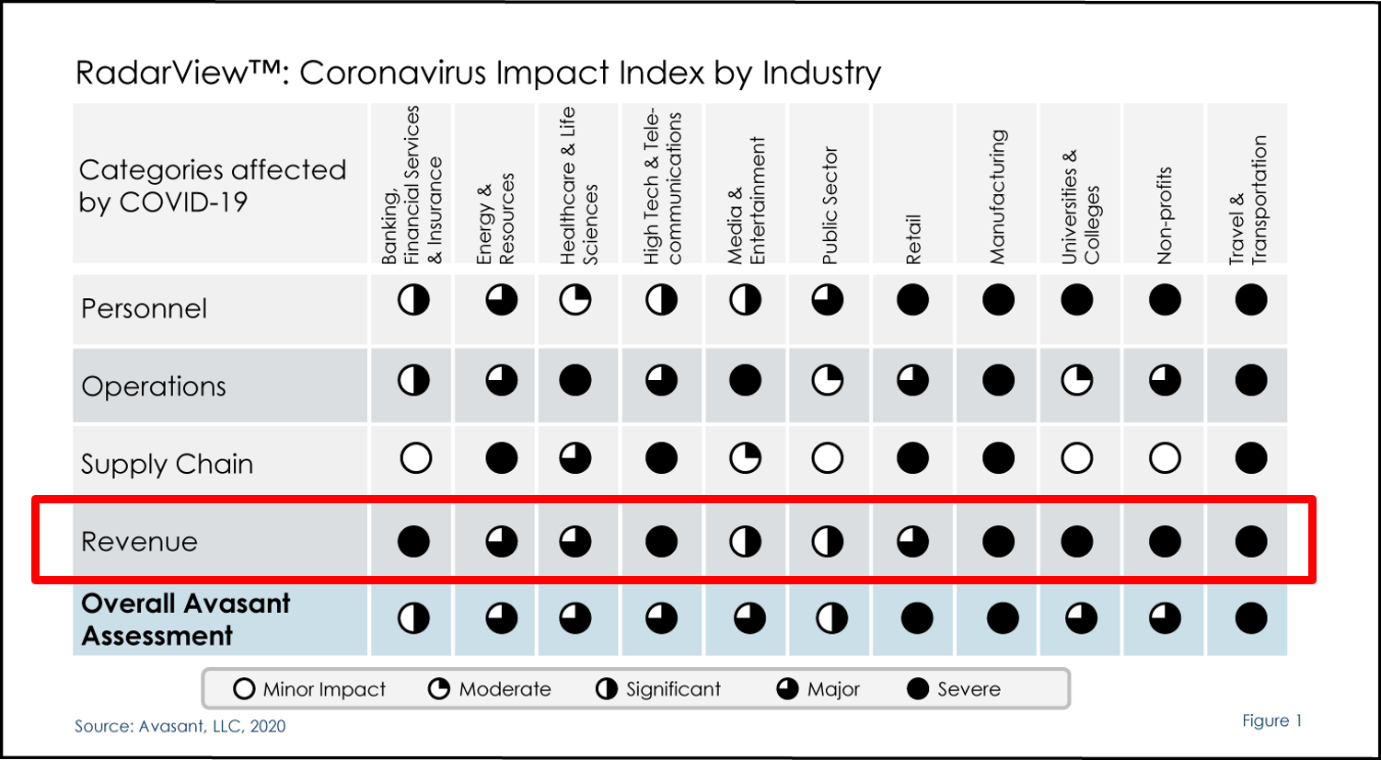
Covid-19 has heavily impacted revenue across industries.Source: Avasant
Companies must focus on three critical business priorities in a post-pandemic economy: customer insights, cost efficiency, and risk reduction. Any AI project must satisfy at least one of these priorities. These priorities will be common across industries in 2020 (at least) since most companies have lost significant revenue.
Customer insights will help firms understand and predict changing customer behavior. While it may not help cut costs today, it will bring new customers and capture market share. Customer insights help firms develop products that customers need and engage in targeted marketing. Establishing this foundation will give firms a sizable lead over competitors when the economy recovers.
Cost efficiency makes perfect sense when revenues are down. AI can help cut costs by automating document analysis, claims processing, and other manual business processes.
Risk reduction is important during economic downturns. Exposure to fraud risk in banking and insurance, for example, increases potential costs. Regulators love to slap billion-dollar fines on banks that fail to prevent fraud and money laundering. These risks must be avoided when revenues are down.
Notable firms have been using AI for these purposes for years now. Business leaders in charge of AI adoption can use these examples to inform their own AI strategy.
JPMorgan Chase: Automating Document Analysis with Natural Language Processing
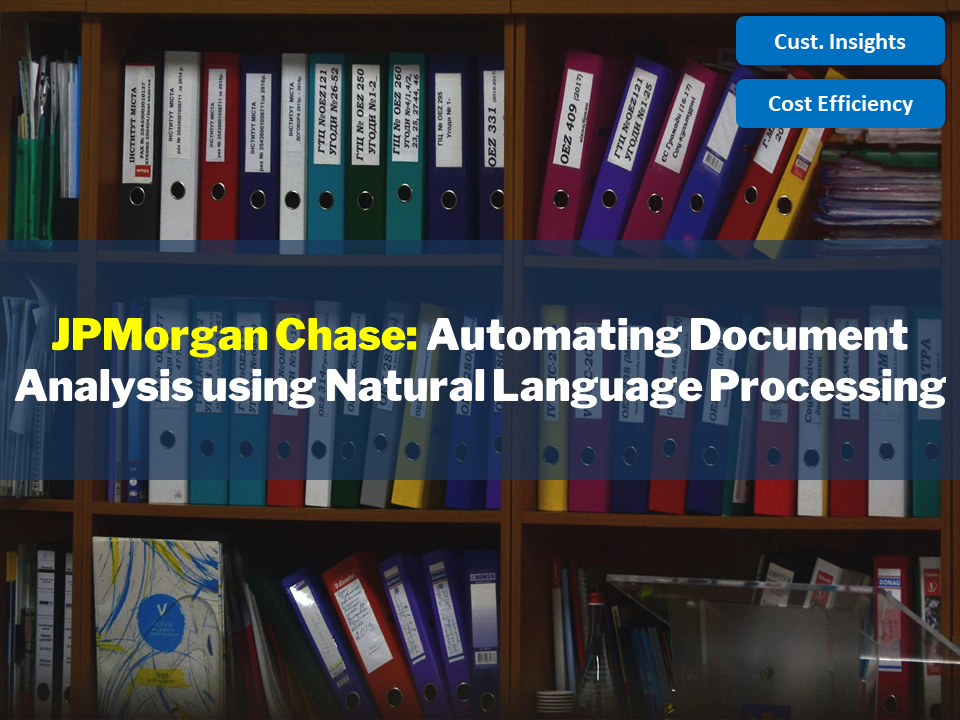
Corporations have teams dedicated to extracting and analyzing information from invoices, customer forms, legal contracts, etc. Most firms have too much data. For example, most insurance companies only process 10–15 percent of their data, according to Accenture. AI can help with this.
JPMorgan rolled out an AI document search tool called COIN (short for Contract Intelligence). COIN helps JPMorgan’s legal department search through thousands of legal documents and extract relevant data in seconds. A lot of legal work involves document analysis to study legal precedents, so any tool that speeds this up is valuable.
COIN is powered by an AI technique called Natural Language Processing (NLP). NLP enables computers to read and interpret text much faster than humans. COIN allegedly extracted 150 types of data that the legal team was interested in from 12,000 commercial credit agreements in seconds. COIN allegedly saves the bank 15,000 days of manual work per year.
NLP and machine learning train software to deal with different document structures and layouts. Traditional software can extract info from a document if the layout and format do not change. Since document formats do change in the real world, AI enables software to ‘read’ and ‘interpret’ many documents types without being programmed to deal with individual cases.
AI tools that interpret documents also help firms generate customer insights. These tools can analyze customer databases and generate a 360-degree customer view, which enables targeted marketing and cross-selling opportunities.
Banks’ investment desks can automate financial report analysis, freeing up human analysts to focus on more complex work. Annual reports are hundreds of pages long. If an AI tool can perform the preliminary analysis, the human analyst can focus on more high-value analysis.
Danske Bank: Improving Fraud Detection with Deep Learning
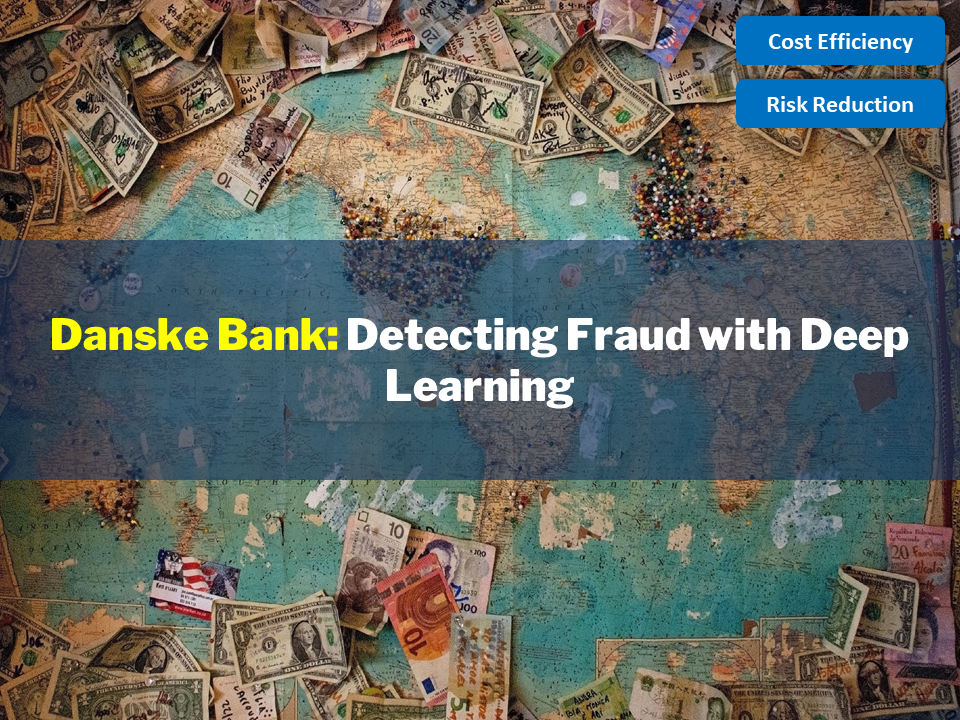
Fraud increases during tough economic times. Banks and insurance companies will see a spike in potential fraudulent transactions that must be investigated. This is incredibly time-consuming. AI tools can detect fraud better and deal with common fraud cases without human intervention, which frees up staff to focus on high-value and complex cases.
Danske Bank is Denmark’s largest bank and they partnered with an analytics firm called Teradata to build a fraud detection system powered by deep learning.
The new system enabled Danske Bank to significantly reduce risks related to fraud. The bank’s fraud detection capability increased by 50% and false positives fell by 60%. Staff can now devote more time to complex and high-value fraud cases.
The bank’s old rules-based system only detected fraud correctly 40% of the time, which exposed the bank to huge risks. The old system also threw up 1,200 false positives per day, where legitimate transactions were incorrectly classified as fraud. Staff wasted a lot of time investigating innocent transactions.
Deep learning sounds complex, but we can think of it is an advanced pattern detection and classification tool. When a deep learning system is fed thousands of transactions, it learns to identify which transactions are ‘normal’ and which are potentially fraudulent. It does this largely without human supervision and improves as it sees more data.
Danske Bank’s new deep learning system consists of different fraud detection models that ‘compete’ with each other to get better at detecting fraud. The winning models train the weaker models, which ensures that all models get better over time.
Ant Financial: Automating Insurance Claims with Machine Vision
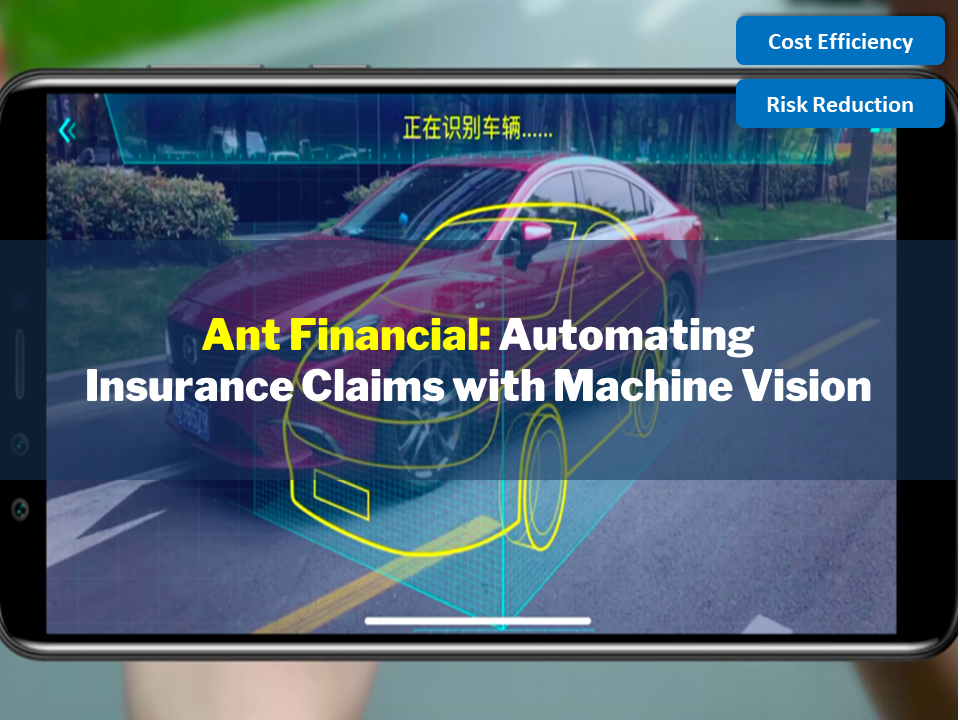
Insurers make money from selling insurance but spend a lot of time and money processing claims. The claims process can be long and painful for both insurers and customers.
A great example is car insurance claims. Normally, if your car gets damaged, you must submit paperwork, maybe talk to a claims agent, and wait a long time to get your money. This process costs the insurance company money too.
Ant Financial, the fintech arm of Alibaba Group, built a mobile app called Dingsunbao that automatically detects damage to your car and instantly pays your claim. Car owners simply take pictures of their cars using the app.
The app uses an AI technique called machine vision to detect objects. Neural networks are also used for image recognition to classify the car type, car part, and type of damage to the car. The app estimates repair costs and determines the impact on the driver’s future insurance premiums. All of this allegedly happens in six seconds.
Automating HR Processes with Intelligent RPA
Companies are turning to AI-enabled Robotic Process Automation (RPA) to automate business processes more effectively. Vendors such as Automation Anywhere provide AI-powered RPA solutions to multiple industries.
According to an Automation Anywhere case study, a global bank used an AI-enabled RPA tool called IQ Bot to automate numerous Human Resources functions and save $1M annually.
The bank’s HR department manages hundreds of HR onboarding forms across languages and countries. HR staff used to manually sort, manage, and process these forms and manually enter data into the HR Management System. This approach was slow and error-prone.
Automation Anywhere’s IQ Bot was able to process these forms and take care of data entry. First, task bots downloaded the forms and fed them into IQ Bot. Next, IQ Bot extracted and cleaned relevant data from the forms and automatically entered records into the bank’s HR Management System.
Takeaways for Business Leaders
The post-coronavirus economy will be challenging and uncertain. Companies will prioritize generating revenue through customer insights, cutting costs, and lowering risk.
When it comes to AI projects, companies can look at low-cost and high-ROI project in the short term. How corporate leaders choose AI projects depends on their company’s financial situation and business needs. Executives must understand their business needs and challenges, and then select AI tools that meet these needs.
Successful AI projects need close collaboration between tech and business teams. Business leaders and functional experts must be able to translate business requirements into technical requirements. This ensures that AI tools can meet current (and evolving) business needs.
A staff that understands how the business operates and has AI awareness is well suited to act as a bridge between the business and tech side. They will also be able to effectively use AI tools after they are built. Executives should select these employees across departments to manage AI projects and adoption.
Contact us at info@blu.ltd to discuss how your company can identify & implement high-value AI solutions. Feel free to contact our management team directly as well.
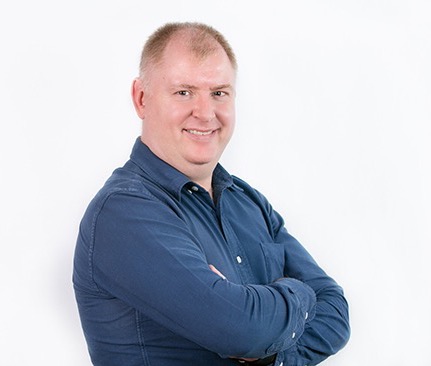
Fabrice Fischer
CEO
fabrice@blu.ltd
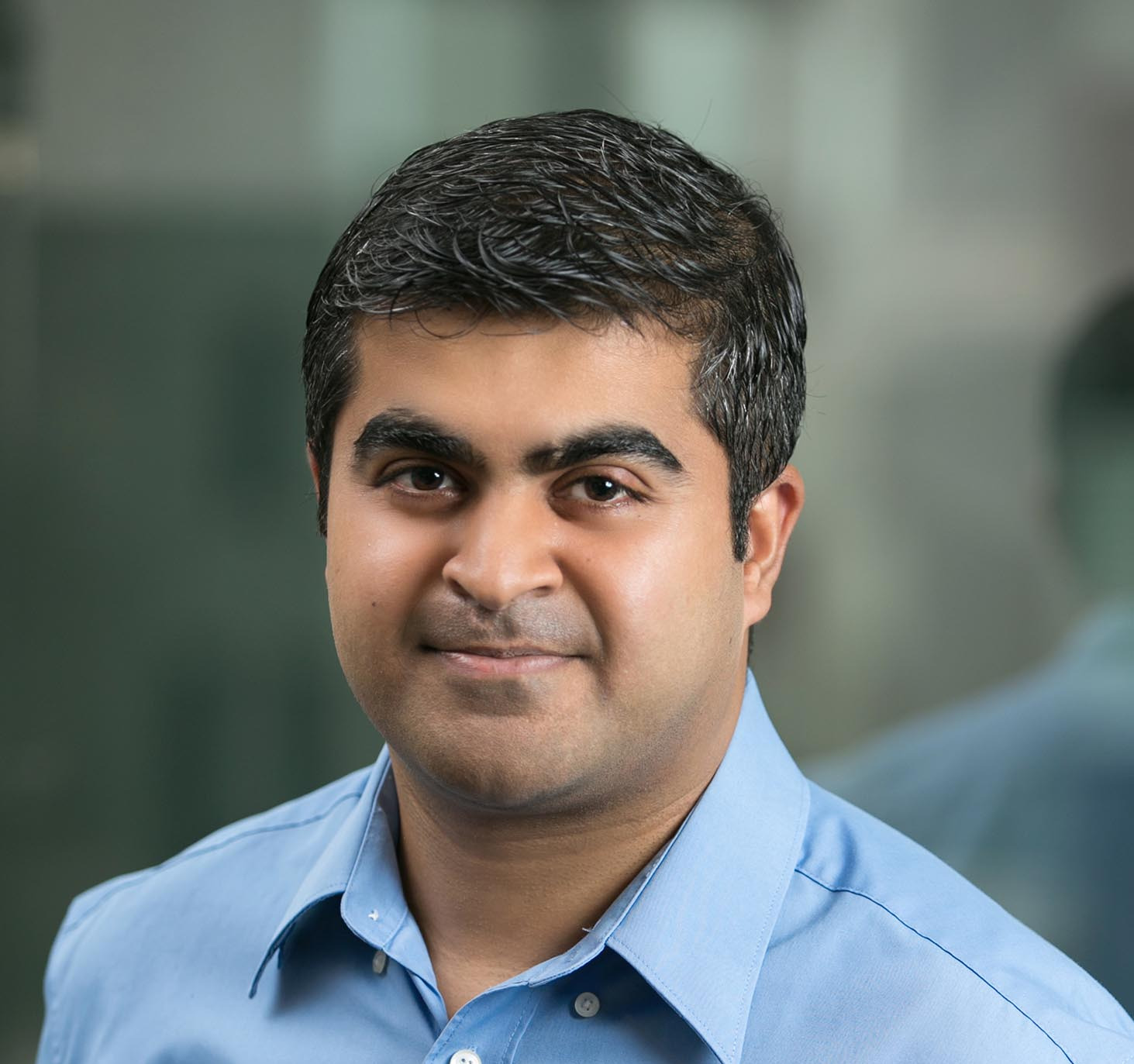
Kevin Pereira
Managing Director, Financial Services
kevin@blu.ltd
About the Author
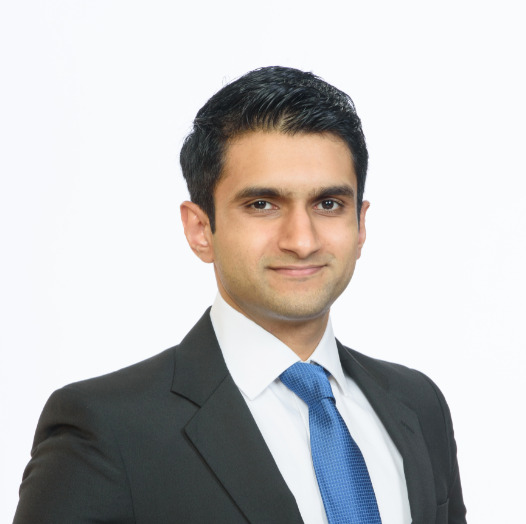
Rajendra Shroff
Manager
rajendra.shroff@blu.ltd
Raj Shroff is an AI Consultant with a background in Insurance. Prior to joining Blu, he was with AXA in Hong Kong.